Detecting Similarities in Vectorized Documents
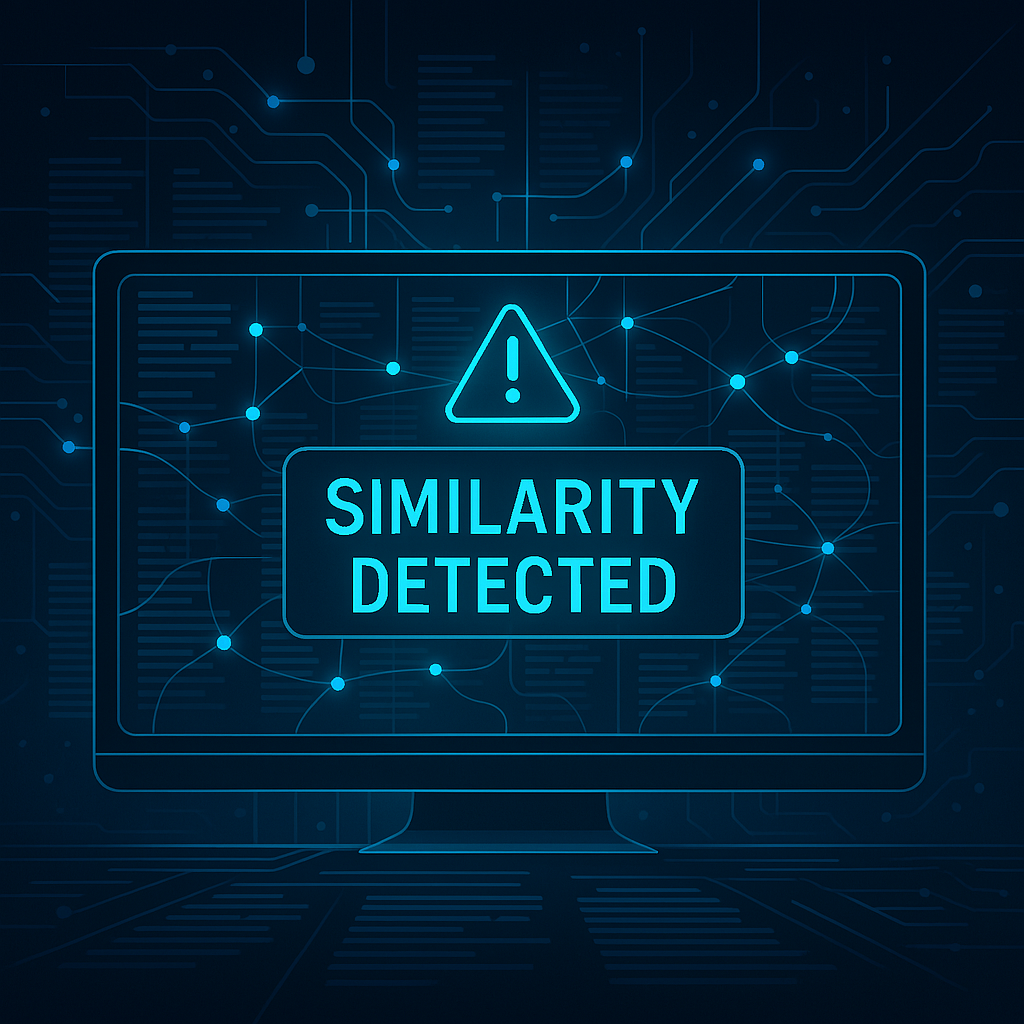
One particularly useful technique when working with vectorized documents is similarity detection—in simpler terms, identifying when an author may have copied or heavily borrowed from another source. Whether it's a literal copy/paste from another document or paraphrased content, semantic embeddings allow us to catch it.
How Similar is "Too Similar"?
The degree of similarity between two text chunks is expressed as a number—typically, the closer the number is to -1, the more similar the texts are. What's great is that to perform this kind of search, you don't need AI at all. A simple SQL SELECT will do the trick.
Here's an example:
SELECT a.cluster AS cluster_a,
a.file AS file_a,
a.page AS page_a,
a.position AS position_a,
b.cluster AS cluster_b,
b.file AS file_b,
b.page AS page_b,
b.position AS position_b,
a.embedding <#> b.embedding AS similarity,
a.text_chunk AS text_chunk_a,
b.text_chunk AS text_chunk_b
FROM embeddings a, embeddings b
WHERE a.id < b.id
ORDER BY similarity ASC
LIMIT 20;
This query surfaces the top 20 most similar text pairs in your dataset, based on vector distance.
Example of the this SELECT operation applied to the documents mentioned in the previous blog post.
cluster_a | file_a | page_a | position_a | cluster_b | file_b | page_b | position_b | similarity |
---|---|---|---|---|---|---|---|---|
EU Budget Appropriations | SEC03.pdf | 831 | 1 | EU Budget Appropriations | SEC03.pdf | 825 | 1 | -0.9989223480224609 |
Draft Budget 2025 | SEC03.pdf | 1585 | 1 | Draft Budget 2025 | SEC03.pdf | 1431 | 1 | -0.992438793182373 |
Draft Budget 2025 | SEC03.pdf | 1587 | 1 | Draft Budget 2025 | SEC03.pdf | 1433 | 1 | -0.9922237992286682 |
EU Regulations and Agencies | SEC03.pdf | 231 | 4 | EU Regulations and Agencies | SEC03.pdf | 241 | 3 | -0.9897857904434204 |
EU Budget Appropriations | SEC03.pdf | 1041 | 2 | EU Budget Appropriations | SEC03.pdf | 1017 | 2 | -0.9887492656707764 |
Draft Budget 2025 | SEC03.pdf | 1589 | 1 | Draft Budget 2025 | SEC03.pdf | 1435 | 1 | -0.9879744648933411 |
EU Budget Overview | SEC00.pdf | 3 | 1 | EU Budget Overview | GenExp.pdf | 3 | 1 | -0.9874448776245117 |
Draft Budget 2025 | SEC09.pdf | 7 | 1 | Draft Budget 2025 | SEC08.pdf | 7 | 1 | -0.986766517162323 |
EU Budget Overview | SEC03.pdf | 639 | 1 | EU Budget Overview | SEC03.pdf | 297 | 1 | -0.9862082004547119 |
Draft Budget 2025 | SEC03.pdf | 1439 | 1 | Draft Budget 2025 | SEC03.pdf | 1593 | 1 | -0.9858852624893188 |
EU Budget Appropriations | SEC02.pdf | 67 | 1 | EU Budget Appropriations | SEC10.pdf | 65 | 1 | -0.985404908657074 |
Draft Budget 2025 | SEC07.pdf | 9 | 1 | Draft Budget 2025 | SEC01.pdf | 11 | 1 | -0.984980583190918 |
Draft Budget 2025 | SEC02.pdf | 9 | 2 | Draft Budget 2025 | SEC10.pdf | 9 | 2 | -0.9844917058944702 |
EU Budget Appropriations | SEC10.pdf | 9 | 1 | EU Budget Appropriations | SEC07.pdf | 11 | 1 | -0.9843403100967407 |
EU Budget Appropriations | SEC03.pdf | 1091 | 2 | EU Budget Appropriations | SEC03.pdf | 1041 | 2 | -0.9831921458244324 |
Draft Budget 2025 | SEC03.pdf | 1445 | 1 | Draft Budget 2025 | SEC03.pdf | 1599 | 1 | -0.9828660488128662 |
European Strategic Investments | SEC03.pdf | 917 | 3 | European Strategic Investments | SEC03.pdf | 915 | 3 | -0.9826010465621948 |
Draft Budget 2025 | SEC02.pdf | 11 | 1 | Draft Budget 2025 | SEC10.pdf | 11 | 1 | -0.9824360609054565 |
EU Regulations and Agencies | SEC03.pdf | 479 | 3 | EU Regulations and Agencies | LR01.pdf | 115 | 6 | -0.9824023842811584 |
EU Budget Overview | SEC02.pdf | 1 | 1 | EU Budget Overview | SEC01.pdf | 1 | 1 | -0.9817304611206055 |
When Does Similarity Matter?
The importance of these findings depends on the nature of your documents. For example:
-
In financial reports or structured tables, a high number of similar passages might be perfectly normal.
-
In academic papers or theses, it could be a red flag for plagiarism.
Zooming In: Similarity Within Clusters
You can refine your approach further by restricting similarity checks to within the same cluster. Here's how to count how many high-similarity text pairs exist per cluster:
SELECT
a.cluster,
COUNT(*) AS count_above_threshold
FROM embeddings a
JOIN embeddings b ON a.id < b.id AND a.cluster = b.cluster
WHERE a.embedding <#> b.embedding < -0.98 -- set your threshold GROUP BY a.cluster
ORDER BY count_above_threshold DESC;
Example of the this SELECT operation applied to the documents mentioned in the previous blog post.
cluster | count_above_threshold |
---|---|
Draft Budget 2025 | 12 |
EU Budget Appropriations | 7 |
EU Budget Overview | 3 |
EU Regulations and Agencies | 2 |
European Strategic Investments | 1 |
This helps you identify clusters with unusually high internal similarity—potentially indicating redundancy or heavy reuse of content.
No AI Needed
These examples highlight how you can work with vectorized text data without invoking AI models for every query. It's a fast, transparent, and surprisingly powerful approach.
If you have ideas, use cases, or questions, I'd love to hear from you.
Happy text mining! 🧠📊
Would you like me to add visuals or code snippets for sharing on LinkedIn too?